A Novel Detector of Atypical Beats for Early Diagnosis of Heart Diseases Based on the Stacked Beats Representation of 12-lead ECG
DOI:
https://doi.org/10.17488/RMIB.44.4.6Keywords:
atypical beats detection, cardiovascular diseases at an early stage, ECG computer-assisted analysis, preventive medicine, stacked beats representation of ECGAbstract
We have developed and present in this work a series of algorithms that present a long-duration electrocardiogram (ECG) in a compact form of stacked beats, extracting and visualizing the basic features and facilitating the tedious and time-consuming process of ECG analysis for cardiologists. The expert system based on this representation provides detection of atypical heartbeats, precursors of cardiovascular disease, and their locations in each of the 12 leads. This system was extensively tested with two public databases, MIT-BIH arrhythmia database and China Physiological Signal Challenge (CPSC2018), showing its rapid ECG processing and high efficiency in detecting abnormalities in beat morphology. In particular, tests for atypical beats based on the CPSC2018 database revealed that the set of ECGs marked as normal contains a considerable number of leads with atypical beats. The system is used as a classifier into two classes, normal beats, and atypical beats, the latter being the precursors or indicators of cardiovascular diseases (CVD). It is considered potentially useful for routine studies in groups at high risk of CVD in early stages, as a preventive medicine tool in the public health area. The system allows an intervention of a cardiologist in the intermediate stages of ECG analysis to corroborate the diagnosis in ambiguous cases.
Downloads
References
C. K. Jha & M. H. Kolekar, “Empirical Mode Decomposition and Wavelet Transform Based ECG Data Compression Scheme,” IRBM, vol. 42, no. 1, pp. 65–72, Feb. 2021, doi: https://doi.org/10.1016/j.irbm.2020.05.008
B. Mohamed, A. Issam, A. Mohamed, & B. Abdellatif, “ECG Image Classification in Real time based on the Haar-like Features and Artificial Neural Networks,” Procedia Comput. Sci., vol. 73, pp. 32–39, 2015, doi: https://doi.org/10.1016/j.procs.2015.12.045
I. Güler & E. D. Übeyli, “ECG beat classifier designed by combined neural network model,” Pattern Recognit., vol. 38, no. 2, pp. 199–208, Feb. 2005, doi: https://doi.org/10.1016/j.patcog.2004.06.009
L.-H. Wang, Y.-T. Yu, W. Liu, L. Xu, et al., “Three-Heartbeat Multilead ECG Recognition Method for Arrhythmia Classification,” IEEE Access, vol. 10, pp. 44046-44061, Apr. 2022, doi: https://doi.org/10.1109/ACCESS.2022.3169893
Z. Dokur & T. Ölmez, “ECG beat classification by a novel hybrid neural network,” Comput. Methods Programs Biomed., vol. 66, no. 2–3, pp. 167-181, Sep. 2001, doi: https://doi.org/10.1016/S0169-2607(00)00133-4
J.-S. Wang, W.-C. Chiang, Y.-L. Hsu, Y.-T. C. Yang, “ECG arrhythmia classification using a probabilistic neural network with a feature reduction method,” Neurocomputing, vol. 116, pp. 38-45, Sep. 2013, doi: https://doi.org/10.1016/j.neucom.2011.10.045
S. M. Anwar, M. Gul, M. Majid, M. Alnowami, “Arrhythmia Classification of ECG Signals Using Hybrid Features,” Comput. Math. Methods Med., vol. 2018, art. no. 1380348, 2018, doi: https://doi.org/10.1155/2018/1380348
F. A. Elhaj, N. Salim, A. R. Harris, T. T. Swee, T. Ahmed, “Arrhythmia recognition and classification using combined linear and nonlinear features of ECG signals,” Comput. Methods Programs Biomed., vol. 127, pp. 52-63, Apr. 2016, doi: https://doi.org/10.1016/j.cmpb.2015.12.024
M. K. Moridani, M. Abdi Zadeh, Z. Shahiazar Mazraeh, “An Efficient Automated Algorithm for Distinguishing Normal and Abnormal ECG Signal,” IRBM, vol. 40, no. 6, pp. 332-340, Dec. 2019, doi: https://doi.org/10.1016/j.irbm.2019.09.002
S. Sahoo, M. Mohanty, S. Behera, & S. Kumar Sabut, “ECG beat classification using empirical mode decomposition and mixture of features”, J. Med. Eng. Technol., vol. 41, no. 8, pp. 652-661, 2017, doi: https://doi.org/10.1080/03091902.2017.1394386
Ö. Yildirim, “A novel wavelet sequence based on deep bidirectional LSTM network model for ECG signal classification,” Comput. Biol. Med., vol. 96, pp. 189-202, May 2018, doi: https://doi.org/10.1016/j.compbiomed.2018.03.016
R. A. Alharbey, S. Alsubhi, K. Daqrouq, A. Alkhateeb, “The continuous wavelet transform using for natural ECG signal arrhythmias detection by statistical parameters,” Alex. Eng. J., vol. 61, no. 12, pp. 9243-9248, Dec. 2022, doi: https://doi.org/10.1016/j.aej.2022.03.016
J. Park & K. Kang, “PcHD: Personalized classification of heartbeat types using a decision tree,” Comput. Biol. Med., vol. 54, pp. 79-88, Nov. 2014, doi: https://doi.org/10.1016/j.compbiomed.2014.08.013
Y. Kutlu & D. Kuntalp, “A multi-stage automatic arrhythmia recognition and classification system,” Comput. Biol. Med., vol. 41, no. 1, pp. 37-45, Jan. 2011, doi: https://doi.org/10.1016/j.compbiomed.2010.11.003
J. Kim & H. Shin, “Simple and Robust Realtime QRS Detection Algorithm Based on Spatiotemporal Characteristic of the QRS Complex,” PLoS ONE, vol. 11, no. 3, art. no. e0150144, 2016, doi: https://doi.org/10.1371/journal.pone.0150144
N. Katsaouni, F. Aul, L. Krischker, S. Schmalhofer, L. Hedrich, M. H. Schulz, “Energy efficient convolutional neural networks for arrhythmia detection,” Array, vol. 13, art. no. 100127, 2022, doi: https://doi.org/10.1016/j.array.2022.100127
X. Yang, X. Zhang, M. Yang, L. Zhang, “12-Lead ECG arrhythmia classification using cascaded convolutional neural network and expert feature,” J. Electrocardiol., vol. 67, pp. 56-62, 2021, doi: https://doi.org/10.1016/j.jelectrocard.2021.04.016
S. G. Begum, E. Priyadarshi, S. Pratap, S. Kulshrestha, V. Singh, “Automated Detection of Abnormalities in ECG signals using Deep Neural Network,” Biomed. Eng. Adv., vol. 5, art. no. 100066, Jun. 2023, doi: https://doi.org/10.1016/j.bea.2022.100066
S. C. Mohonta, M. A. Motin, & D. K. Kumar, “Electrocardiogram Based Arrhythmia Classification Using Wavelet Transform with Deep Learning Model,” Sens. Bio-Sens. Res., vol. 37, 2022, doi: http://dx.doi.org/10.2139/ssrn.4088025
J. H. Abawajy, A.V. Kelarev, M. Chowdhury, “Multistage approach for clustering and classification of ECG data,” Comput. Methods Programs Biomed., vol. 112, no. 3, pp. 720-730, Dec. 2013, doi: https://doi.org/10.1016/j.cmpb.2013.08.002
W.-H. Jung & S.-G. Lee, “An Arrhythmia Classification Method in Utilizing the Weighted KNN and the Fitness Rule,” IRBM, vol. 38, no. 3, pp. 138-148, Jun. 2017, doi: https://doi.org/10.1016/j.irbm.2017.04.002
L. Lu, J. Yan, C. W. de Silva, “Feature selection for ECG signal processing using improved genetic algorithm and empirical mode decomposition,” Measurement, vol. 94, pp. 372-381, Dec. 2016, doi: https://doi.org/10.1016/j.measurement.2016.07.043
U. R. Acharya, P. Subbanna Bhat, S.S. Iyengar, A. Rao, Sumeet Dua, “Classification of heart rate data using artificial neural network and fuzzy equivalence relation,” Pattern Recognit., vol. 36, no. 1, pp. 61-68, Jan. 2003, doi: https://doi.org/10.1016/S0031-3203(02)00063-8
J. Rahul, M. Sora, L. D. Sharma, V. K. Bohat, “An improved cardiac arrhythmia classification using an RR interval-based approach,” Biocybern. Biomed. Eng., vol. 41, no. 2, pp. 656-666, 2021, doi: https://doi.org/10.1016/j.bbe.2021.04.004
R. Rohmantri & N. Surantha, “Arrhythmia Classification using 2D Convolutional Neural Network,” Int. J. Adv. Comput. Sci. Appl., vol. 11, no. 4, 2020, doi: http://dx.doi.org/10.14569/IJACSA.2020.0110427
S. L. Oh, E. Y. K. Ng, R. S. Tan, U. R. Acharya, Automated diagnosis of arrhythmia using combination of CNN and LSTM techniques with variable length heart beats, Comput. Biol. Med., vol. 102, pp. 278-287, Nov. 2018, doi: https://doi.org/10.1016/j.compbiomed.2018.06.002
B. Chen, D. M. Maslove, J. D. Curran, A. Hamilton, P. R. Laird, P. Mousavi, S. Sibley, “A deep learning model for the classification of atrial fibrillation in critically ill patients,” Intensive Care Med. Exp., vol. 11, art. no. 2, Jan. 2023, doi: https://doi.org/10.1186/s40635-022-00490-3
O. Yildirim, U. B. Baloglu, R.-S. Tan, E. J. Ciaccio, U. R. Acharya, “A new approach for arrhythmia classification using deep coded features and LSTM networks,” Comput. Methods Programs Biomed., vol. 176, pp. 121-133, Jul. 2019, doi: https://doi.org/10.1016/j.cmpb.2019.05.004
N. Du, Q. Cao, L. Yu, N. Liu, E. Zhong, Z. Liu, Y. Shen, K. Chen, Fm-ecg: A fine-grained multi-label framework for ecg image classification, Information Sciences 549 (2021) pp. 164–177, doi: https://doi.org/10.1016/j.ins.2020.10.014
A. Aranda Hernandez, P. Bonizzi, R. Peeters, J. Karel, “Continuous monitoring of acute myocardial infarction with a 3-Lead ECG system,” Biomed. Signal Process. Control, vol. 79, art. no. 104041, Jan. 2023, doi: https://doi.org/10.1016/j.bspc.2022.104041
G. B. Moody & R. G. Mark, “The impact of the MIT-BIH Arrhythmia Database,” IEEE Eng. Med. Biol. Mag., vol. 20, no. 3, pp. 45-50, 2001, doi: https://doi.org/10.1109/51.932724
F. Liu, C. Liu, L. Zhao, X. Zhang, et al., “An Open Access Database for Evaluating the Algorithms of Electrocardiogram Rhythm and Morphology Abnormality Detection,” J. Med. Imaging Health Inf., vol. 8, no. 7, pp. 1368–1373, 2018, doi: http://dx.doi.org/10.1166/jmihi.2018.2442
M. Bellamoli, F. Marin, L. Maritan, D. Prati, et al., “New-onset extreme right axis deviation in acute myocardial infarction: clinical characteristics and outcomes,” J. Electrocardiol., vol 60, pp. 60–66, 2020, doi: https://doi.org/10.1016/j.jelectrocard.2020.03.003
X. Gu, J. Hu, L. Zhang, J. Ding, F. Yan, “An Improved Method with High Anti-interference Ability for R Peak Detection in Wearable Devices,” IRBM, vol. 41, no. 3, pp. 172–183, Jun. 2020, doi: https://doi.org/10.1016/j.irbm.2020.01.002
C. Cakir, Y. Ceylan, O. Y. Akbal, R. Sarikaya, S. Barutcu, “Clinical characteristics and angiographic findings of non-st-elevation acute coronary syndrome patients admitted with normal electrocardiogram,” J. Electrocardiol., vol. 60, pp. 77–81, 2020, doi: https://doi.org/10.1016/j.jelectrocard.2020.03.002
N. Dugarte, E. Dugarte, N. Dugarte, Electrocardiografía de Alta Resolución Técnicas Aplicadas de Adquisición y Procesamiento, Argentina: Universidad Tecnológica Nacional (UTN-FRM), 2018.
R. Srivastva, A. Singh, Y. N. Singh, “PlexNet: A fast and robust ECG biometric system for human recognition,” Information Sciences, vol. 558, pp. 208-228, May 2021, doi: https://doi.org/10.1016/j.ins.2021.01.001
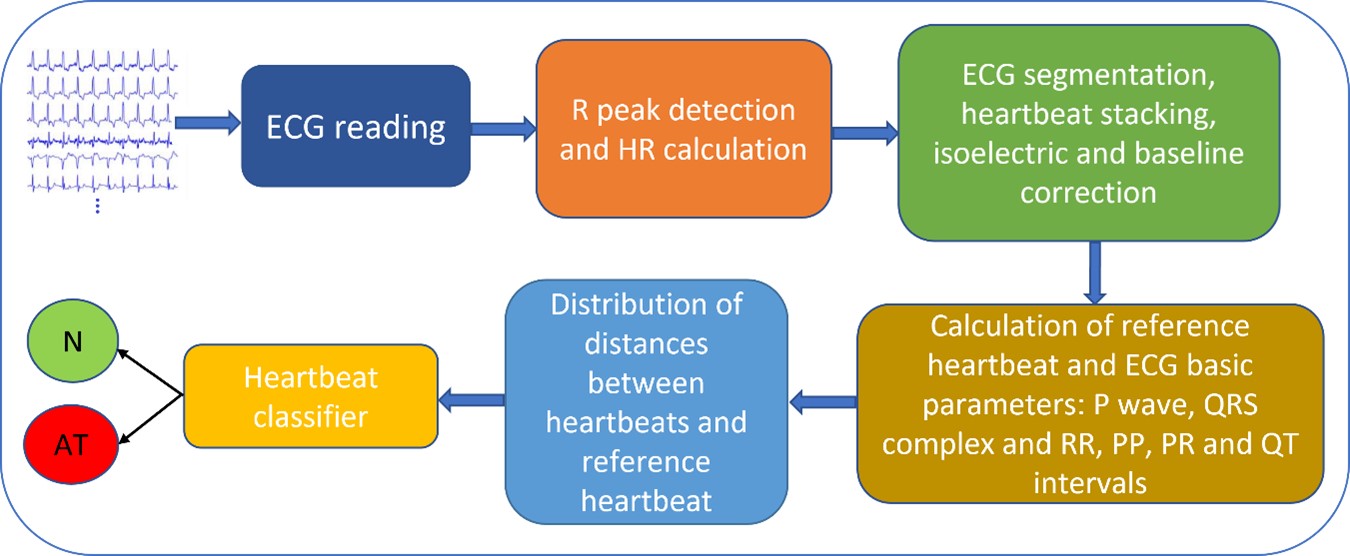
Published
How to Cite
Issue
Section
License
Copyright (c) 2023 Revista Mexicana de Ingenieria Biomedica

This work is licensed under a Creative Commons Attribution-NonCommercial 4.0 International License.
Upon acceptance of an article in the RMIB, corresponding authors will be asked to fulfill and sign the copyright and the journal publishing agreement, which will allow the RMIB authorization to publish this document in any media without limitations and without any cost. Authors may reuse parts of the paper in other documents and reproduce part or all of it for their personal use as long as a bibliographic reference is made to the RMIB. However written permission of the Publisher is required for resale or distribution outside the corresponding author institution and for all other derivative works, including compilations and translations.